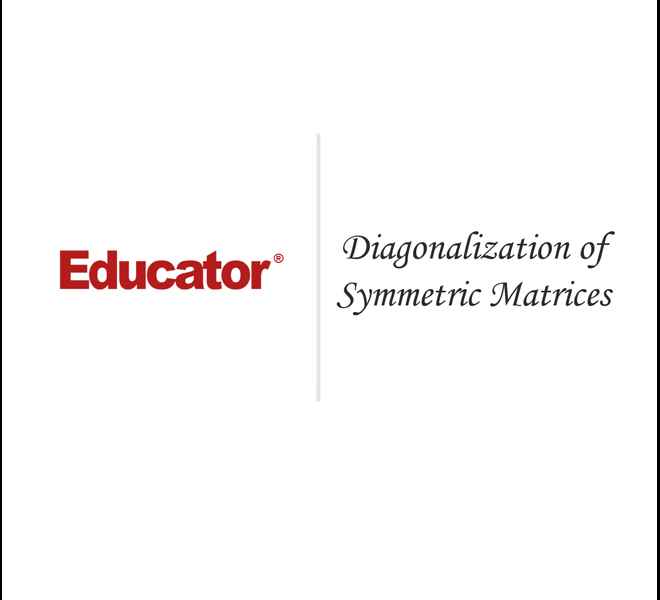
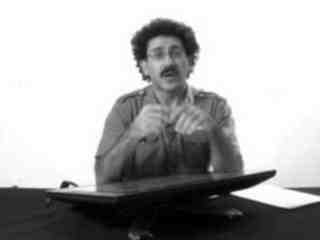
Raffi Hovasapian
Diagonalization of Symmetric Matrices
Slide Duration:Table of Contents
39m 3s
- Intro0:00
- Linear Systems1:20
- Introduction to Linear Systems1:21
- Examples10:35
- Example 110:36
- Example 213:44
- Example 316:12
- Example 423:48
- Example 528:23
- Example 632:32
- Number of Solutions35:08
- One Solution, No Solution, Infinitely Many Solutions35:09
- Method of Elimination36:57
- Method of Elimination36:58
30m 34s
- Intro0:00
- Matrices0:47
- Definition and Example of Matrices0:48
- Square Matrix7:55
- Diagonal Matrix9:31
- Operations with Matrices10:35
- Matrix Addition10:36
- Scalar Multiplication15:01
- Transpose of a Matrix17:51
- Matrix Types23:17
- Regular: m x n Matrix of m Rows and n Column23:18
- Square: n x n Matrix With an Equal Number of Rows and Columns23:44
- Diagonal: A Square Matrix Where All Entries OFF the Main Diagonal are '0'24:07
- Matrix Operations24:37
- Matrix Operations24:38
- Example25:55
- Example25:56
41m 42s
- Intro0:00
- Dot Product1:04
- Example of Dot Product1:05
- Matrix Multiplication7:05
- Definition7:06
- Example 112:26
- Example 217:38
- Matrices and Linear Systems21:24
- Matrices and Linear Systems21:25
- Example 129:56
- Example 232:30
- Summary33:56
- Dot Product of Two Vectors and Matrix Multiplication33:57
- Summary, cont.35:06
- Matrix Representations of Linear Systems35:07
- Examples35:34
- Examples35:35
43m 17s
- Intro0:00
- Properties of Addition1:11
- Properties of Addition: A1:12
- Properties of Addition: B2:30
- Properties of Addition: C2:57
- Properties of Addition: D4:20
- Properties of Addition5:22
- Properties of Addition5:23
- Properties of Multiplication6:47
- Properties of Multiplication: A7:46
- Properties of Multiplication: B8:13
- Properties of Multiplication: C9:18
- Example: Properties of Multiplication9:35
- Definitions and Properties (Multiplication)14:02
- Identity Matrix: n x n matrix14:03
- Let A Be a Matrix of m x n15:23
- Definitions and Properties (Multiplication)18:36
- Definitions and Properties (Multiplication)18:37
- Properties of Scalar Multiplication22:54
- Properties of Scalar Multiplication: A23:39
- Properties of Scalar Multiplication: B24:04
- Properties of Scalar Multiplication: C24:29
- Properties of Scalar Multiplication: D24:48
- Properties of the Transpose25:30
- Properties of the Transpose25:31
- Properties of the Transpose30:28
- Example30:29
- Properties of Matrix Addition33:25
- Let A, B, C, and D Be m x n Matrices33:26
- There is a Unique m x n Matrix, 0, Such That…33:48
- Unique Matrix D34:17
- Properties of Matrix Multiplication34:58
- Let A, B, and C Be Matrices of the Appropriate Size34:59
- Let A Be Square Matrix (n x n)35:44
- Properties of Scalar Multiplication36:35
- Let r and s Be Real Numbers, and A and B Matrices36:36
- Properties of the Transpose37:10
- Let r Be a Scalar, and A and B Matrices37:12
- Example37:58
- Example37:59
38m 14s
- Intro0:00
- Reduced Row Echelon Form0:29
- An m x n Matrix is in Reduced Row Echelon Form If:0:30
- Reduced Row Echelon Form2:58
- Example: Reduced Row Echelon Form2:59
- Theorem8:30
- Every m x n Matrix is Row-Equivalent to a UNIQUE Matrix in Reduced Row Echelon Form8:31
- Systematic and Careful Example10:02
- Step 110:54
- Step 211:33
- Step 312:50
- Step 414:02
- Step 515:31
- Step 617:28
- Example30:39
- Find the Reduced Row Echelon Form of a Given m x n Matrix30:40
28m 54s
- Intro0:00
- Solutions of Linear Systems0:11
- Solutions of Linear Systems0:13
- Example I3:25
- Solve the Linear System 13:26
- Solve the Linear System 214:31
- Example II17:41
- Solve the Linear System 317:42
- Solve the Linear System 420:17
- Homogeneous Systems21:54
- Homogeneous Systems Overview21:55
- Theorem and Example24:01
40m 10s
- Intro0:00
- Finding the Inverse of a Matrix0:41
- Finding the Inverse of a Matrix0:42
- Properties of Non-Singular Matrices6:38
- Practical Procedure9:15
- Step19:16
- Step 210:10
- Step 310:46
- Example: Finding Inverse12:50
- Linear Systems and Inverses17:01
- Linear Systems and Inverses17:02
- Theorem and Example21:15
- Theorem26:32
- Theorem26:33
- List of Non-Singular Equivalences28:37
- Example: Does the Following System Have a Non-trivial Solution?30:13
- Example: Inverse of a Matrix36:16
21m 25s
- Intro0:00
- Determinants0:37
- Introduction to Determinants0:38
- Example6:12
- Properties9:00
- Properties 1-59:01
- Example10:14
- Properties, cont.12:28
- Properties 6 & 712:29
- Example14:14
- Properties, cont.18:34
- Properties 8 & 918:35
- Example19:21
59m 31s
- Intro0:00
- Cofactor Expansions and Their Application0:42
- Cofactor Expansions and Their Application0:43
- Example 13:52
- Example 27:08
- Evaluation of Determinants by Cofactor9:38
- Theorem9:40
- Example 111:41
- Inverse of a Matrix by Cofactor22:42
- Inverse of a Matrix by Cofactor and Example22:43
- More Example36:22
- List of Non-Singular Equivalences43:07
- List of Non-Singular Equivalences43:08
- Example44:38
- Cramer's Rule52:22
- Introduction to Cramer's Rule and Example52:23
46m 54s
- Intro0:00
- Vectors in the Plane0:38
- Vectors in the Plane0:39
- Example 18:25
- Example 215:23
- Vector Addition and Scalar Multiplication19:33
- Vector Addition19:34
- Scalar Multiplication24:08
- Example26:25
- The Angle Between Two Vectors29:33
- The Angle Between Two Vectors29:34
- Example33:54
- Properties of the Dot Product and Unit Vectors38:17
- Properties of the Dot Product and Unit Vectors38:18
- Defining Unit Vectors40:01
- 2 Very Important Unit Vectors41:56
52m 44s
- Intro0:00
- n-Vectors0:58
- 4-Vector0:59
- 7-Vector1:50
- Vector Addition2:43
- Scalar Multiplication3:37
- Theorem: Part 14:24
- Theorem: Part 211:38
- Right and Left Handed Coordinate System14:19
- Projection of a Point Onto a Coordinate Line/Plane17:20
- Example21:27
- Cauchy-Schwarz Inequality24:56
- Triangle Inequality36:29
- Unit Vector40:34
- Vectors and Dot Products44:23
- Orthogonal Vectors44:24
- Cauchy-Schwarz Inequality45:04
- Triangle Inequality45:21
- Example 145:40
- Example 248:16
48m 53s
- Intro0:00
- Introduction to Linear Transformations0:44
- Introduction to Linear Transformations0:45
- Example 19:01
- Example 211:33
- Definition of Linear Mapping14:13
- Example 322:31
- Example 426:07
- Example 530:36
- Examples36:12
- Projection Mapping36:13
- Images, Range, and Linear Mapping38:33
- Example of Linear Transformation42:02
34m 8s
- Intro0:00
- Linear Transformations1:29
- Linear Transformations1:30
- Theorem 17:15
- Theorem 29:20
- Example 1: Find L (-3, 4, 2)11:17
- Example 2: Is It Linear?17:11
- Theorem 325:57
- Example 3: Finding the Standard Matrix29:09
37m 54s
- Intro0:00
- Lines and Plane0:36
- Example 10:37
- Example 27:07
- Lines in IR39:53
- Parametric Equations14:58
- Example 317:26
- Example 420:11
- Planes in IR325:19
- Example 531:12
- Example 634:18
42m 19s
- Intro0:00
- Vector Spaces3:43
- Definition of Vector Spaces3:44
- Vector Spaces 15:19
- Vector Spaces 29:34
- Real Vector Space and Complex Vector Space14:01
- Example 115:59
- Example 218:42
- Examples26:22
- More Examples26:23
- Properties of Vector Spaces32:53
- Properties of Vector Spaces Overview32:54
- Property A34:31
- Property B36:09
- Property C36:38
- Property D37:54
- Property F39:00
43m 37s
- Intro0:00
- Subspaces0:47
- Defining Subspaces0:48
- Example 13:08
- Example 23:49
- Theorem7:26
- Example 39:11
- Example 412:30
- Example 516:05
- Linear Combinations23:27
- Definition 123:28
- Example 125:24
- Definition 229:49
- Example 231:34
- Theorem32:42
- Example 334:00
33m 15s
- Intro0:00
- A Spanning Set for a Vector Space1:10
- A Spanning Set for a Vector Space1:11
- Procedure to Check if a Set of Vectors Spans a Vector Space3:38
- Example 16:50
- Example 214:28
- Example 321:06
- Example 422:15
17m 20s
- Intro0:00
- Linear Independence0:32
- Definition0:39
- Meaning3:00
- Procedure for Determining if a Given List of Vectors is Linear Independence or Linear Dependence5:00
- Example 17:21
- Example 210:20
31m 20s
- Intro0:00
- Basis and Dimension0:23
- Definition0:24
- Example 13:30
- Example 2: Part A4:00
- Example 2: Part B6:53
- Theorem 19:40
- Theorem 211:32
- Procedure for Finding a Subset of S that is a Basis for Span S14:20
- Example 316:38
- Theorem 321:08
- Example 425:27
24m 45s
- Intro0:00
- Homogeneous Systems0:51
- Homogeneous Systems0:52
- Procedure for Finding a Basis for the Null Space of Ax = 02:56
- Example 17:39
- Example 218:03
- Relationship Between Homogeneous and Non-Homogeneous Systems19:47
35m 3s
- Intro0:00
- Rank of a Matrix1:47
- Definition1:48
- Theorem 18:14
- Example 19:38
- Defining Row and Column Rank16:53
- If We Want a Basis for Span S Consisting of Vectors From S22:00
- If We want a Basis for Span S Consisting of Vectors Not Necessarily in S24:07
- Example 2: Part A26:44
- Example 2: Part B32:10
29m 26s
- Intro0:00
- Rank of a Matrix0:17
- Example 1: Part A0:18
- Example 1: Part B5:58
- Rank of a Matrix Review: Rows, Columns, and Row Rank8:22
- Procedure for Computing the Rank of a Matrix14:36
- Theorem 1: Rank + Nullity = n16:19
- Example 217:48
- Rank & Singularity20:09
- Example 321:08
- Theorem 223:25
- List of Non-Singular Equivalences24:24
- List of Non-Singular Equivalences24:25
27m 3s
- Intro0:00
- Coordinates of a Vector1:07
- Coordinates of a Vector1:08
- Example 18:35
- Example 215:28
- Example 3: Part A19:15
- Example 3: Part B22:26
33m 47s
- Intro0:00
- Change of Basis & Transition Matrices0:56
- Change of Basis & Transition Matrices0:57
- Example 110:44
- Example 220:44
- Theorem23:37
- Example 3: Part A26:21
- Example 3: Part B32:05
32m 53s
- Intro0:00
- Orthonormal Bases in n-Space1:02
- Orthonormal Bases in n-Space: Definition1:03
- Example 14:31
- Theorem 16:55
- Theorem 28:00
- Theorem 39:04
- Example 210:07
- Theorem 213:54
- Procedure for Constructing an O/N Basis16:11
- Example 321:42
21m 27s
- Intro0:00
- Orthogonal Complements0:19
- Definition0:20
- Theorem 15:36
- Example 16:58
- Theorem 213:26
- Theorem 315:06
- Example 218:20
33m 49s
- Intro0:00
- Relations Among the Four Fundamental Vector Spaces Associated with a Matrix A2:16
- Four Spaces Associated With A (If A is m x n)2:17
- Theorem4:49
- Example 17:17
- Null Space and Column Space10:48
- Projections and Applications16:50
- Projections and Applications16:51
- Projection Illustration21:00
- Example 123:51
- Projection Illustration Review30:15
38m 11s
- Intro0:00
- Eigenvalues and Eigenvectors0:38
- Eigenvalues and Eigenvectors0:39
- Definition 13:30
- Example 17:20
- Example 210:19
- Definition 221:15
- Example 323:41
- Theorem 126:32
- Theorem 227:56
- Example 429:14
- Review34:32
29m 55s
- Intro0:00
- Similar Matrices and Diagonalization0:25
- Definition 10:26
- Example 12:00
- Properties3:38
- Definition 24:57
- Theorem 16:12
- Example 39:37
- Theorem 212:40
- Example 419:12
- Example 520:55
- Procedure for Diagonalizing Matrix A: Step 124:21
- Procedure for Diagonalizing Matrix A: Step 225:04
- Procedure for Diagonalizing Matrix A: Step 325:38
- Procedure for Diagonalizing Matrix A: Step 427:02
30m 14s
- Intro0:00
- Diagonalization of Symmetric Matrices1:15
- Diagonalization of Symmetric Matrices1:16
- Theorem 12:24
- Theorem 23:27
- Example 14:47
- Definition 16:44
- Example 28:15
- Theorem 310:28
- Theorem 412:31
- Example 318:00
24m 5s
- Intro0:00
- Linear Mappings2:08
- Definition2:09
- Linear Operator7:36
- Projection8:48
- Dilation9:40
- Contraction10:07
- Reflection10:26
- Rotation11:06
- Example 113:00
- Theorem 118:16
- Theorem 219:20
26m 38s
- Intro0:00
- Kernel and Range of a Linear Map0:28
- Definition 10:29
- Example 14:36
- Example 28:12
- Definition 210:34
- Example 313:34
- Theorem 116:01
- Theorem 218:26
- Definition 321:11
- Theorem 324:28
25m 54s
- Intro0:00
- Kernel and Range of a Linear Map1:39
- Theorem 11:40
- Example 1: Part A2:32
- Example 1: Part B8:12
- Example 1: Part C13:11
- Example 1: Part D14:55
- Theorem 216:50
- Theorem 323:00
33m 21s
- Intro0:00
- Matrix of a Linear Map0:11
- Theorem 11:24
- Procedure for Computing to Matrix: Step 17:10
- Procedure for Computing to Matrix: Step 28:58
- Procedure for Computing to Matrix: Step 39:50
- Matrix of a Linear Map: Property10:41
- Example 114:07
- Example 218:12
- Example 324:31
For more information, please see full course syllabus of Linear Algebra
Linear Algebra Diagonalization of Symmetric Matrices
We’ve talked about similar matrices, identity matrices, and now we’re going to introduce symmetric matrices. Like the matrices introduced before, symmetric matrices have a special property about them, and that is that the matrix is equal to its transpose. This will complete the theorem introduced last time where the eigenvalues of a matrix can produce a diagonal matrix, where the original matrix has to be a symmetric matrix. After this, we’ll move on to the last subject in linear algebra.
Share this knowledge with your friends!
Copy & Paste this embed code into your website’s HTML
Please ensure that your website editor is in text mode when you paste the code.(In Wordpress, the mode button is on the top right corner.)
- - Allow users to view the embedded video in full-size.
7 answers
Wed May 1, 2013 4:41 AM
Post by Matt C on April 28, 2013
Professor Hovasapian
Sorry to spring all these questions on you in one week, but thursday is my final. My professor gave me a matrix and he said that it was diagonalizable. I went through all the steps and I cannot get it to diagonalize. I have the matrix A= [[2,2,-2], [-1,1,2], [0,1,1]], I write all matrix's in column form. He claims that it is diagonalizable, but I have spent a long time trying to figure this out. Lambda = R.
det(A-RI) = -(R-2)(-1+R)^2. R=2, R=1.
(A-1*I)x=0 and I get [ [1,2,-2], [-1,0,2], [0,1,0]], I then subject that to rref. [[1,0,0], [0,1,0],[.5, .5, 0]]. I only have 1 free variable which means the basis is 1, which is less then k. Is there a way where you can quick check if this matrix is Diagonalizable. Like I said I have spent hours on this and I am getting no where.
1 answer
Mon Feb 25, 2013 3:03 AM
Post by Tach M on February 24, 2013
if the polynomial equation of a matrix M has real and distinct roots, Does it mean that M is similar to a diagonal matrix????